Data Labeling in Machine Learning: A Game Changer for Home Services
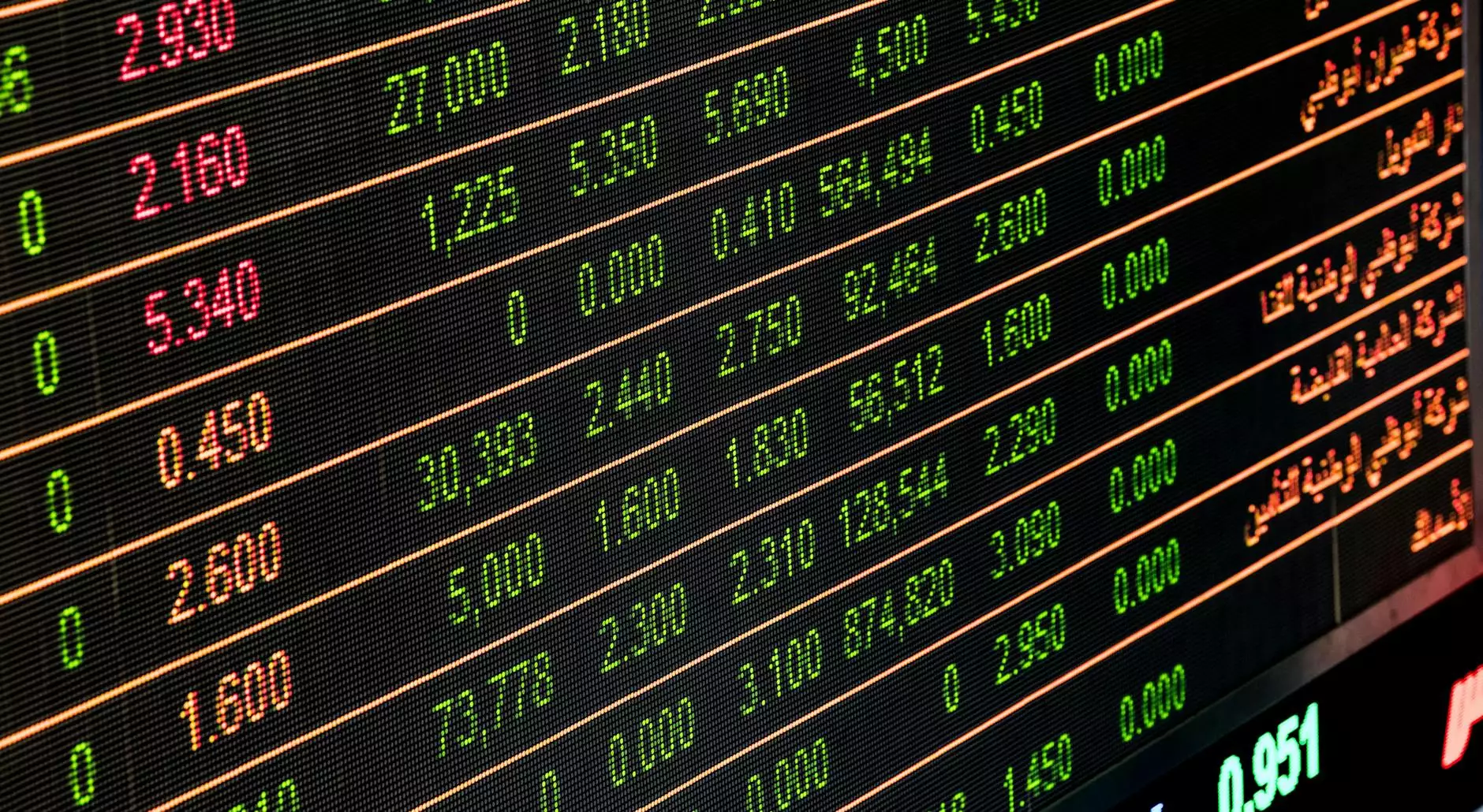
Data labeling is a critical process in machine learning that involves annotating data to enable algorithms to understand it effectively. In the context of businesses like Keymakr, a leading provider of home services and locksmith solutions, the implementation of machine learning through data labeling can enhance operational efficiency, customer service, and decision-making. This article delves deep into the intricacies of data labeling and its essential role in machine learning, particularly for service-based industries.
Understanding Data Labeling
In its simplest form, data labeling involves the identification and tagging of data points that machines need to learn from. This is particularly crucial in supervised learning scenarios, where the model is trained with labeled data to predict outcomes on new, unlabeled data. The labeling can be visual, textual, or auditory and is instrumental in various industries.
The Importance of Data Labeling in Machine Learning
Machine learning models are only as good as the data fed into them. Here's why data labeling holds immense weight:
- Enhanced Model Accuracy: Properly labeled data allows machine learning models to make accurate predictions and decisions, leading to improved outcomes.
- Improved Decision Making: Labeled data enables businesses to leverage insights and analytics for informed decision-making processes.
- Efficient Resource Allocation: By utilizing machine learning, businesses can predict service demands, allowing for optimal resource allocation.
- Customer Satisfaction: Data labeling helps in personalizing customer experiences through predictive analytics.
Application of Data Labeling in Keymakr's Home Services
For a business like Keymakr, specializing in home services and locksmiths, data labeling can be revolutionary. The company can use labeled datasets to:
- Analyze Customer Feedback: By labeling customer reviews and feedback, Keymakr can gauge customer satisfaction and adjust services accordingly.
- Predict Service Trends: Data labeled from historical service requests can help predict peak seasons and service demands based on customer patterns.
- Optimize Marketing Strategies: Labeled data can guide targeted marketing efforts by understanding demographic preferences and behaviors.
- Enhance Service Recommendations: Machine learning algorithms can recommend services based on past customer interactions and preferences.
The Data Labeling Process
The process of data labeling involves several steps, each critical to ensuring the quality and utility of the data. Here’s a detailed breakdown:
1. Data Collection
The first step in the data labeling process is gathering the data that needs to be labeled. This can include customer inquiries, service records, and feedback collected from various channels.
2. Data Preparation
Once data is collected, it must be prepared for labeling. This may involve cleaning the data, removing duplicates, and structuring it in a way that is easy to label.
3. Annotation
Annotation is the actual labeling process. Depending on the type of data, this could involve classifying service requests, tagging keywords in customer feedback, or identifying patterns in service usage.
4. Quality Assurance
After the initial labeling, it’s essential to conduct quality checks to ensure accuracy. This can involve cross-referencing annotations with existing data or conducting reviews by multiple annotators.
5. Integration into Machine Learning Models
Once the data is accurately labeled, it can be integrated into machine learning models for training. This step is crucial for the model's predictive accuracy and overall effectiveness.
Challenges in Data Labeling
While data labeling is undeniably significant, it does come with its challenges:
- Time-Consuming: The process of manually labeling data can be labor-intensive and time-consuming, potentially leading to delays in machine learning deployment.
- Quality Control Issues: Ensuring consistent quality across different datasets can be challenging, especially when multiple annotators are involved.
- Cost Implications: Depending on the extent of the labeling required, costs can escalate, especially if outsourcing labeling services.
Technological Advancements in Data Labeling
Fortunately, technology is continuously evolving to address these challenges. Innovative tools and platforms are emerging to facilitate and optimize the data labeling process:
- Automated Data Labeling: Some machine learning technologies can automatically label data, which can save time and reduce costs.
- Crowdsourcing: Utilizing online platforms to gather multiple annotators can enhance the speed and quality of the data labeling process.
- Labeling Platforms: Specialized platforms like Amazon Mechanical Turk and Labelbox provide streamlined solutions for managing labeling projects efficiently.
How Keymakr Can Leverage Data Labeling
By investing in data labeling, Keymakr can position itself to harness machine learning fully. Here’s how:
- Streamlining Operations: Implementing machine learning models trained on labeled data can automate scheduling, improve response times, and ultimately enhance customer satisfaction.
- Enhancing Security Solutions: Analyzing data patterns can improve Keymakr's locksmith services by predicting security vulnerabilities and adapting solutions accordingly.
- Boosting Customer Retention: By personalizing offers and recommendations through labeled data insights, Keymakr can increase customer loyalty and retention.
The Future of Data Labeling and Machine Learning
As the realm of machine learning continues to evolve, data labeling will remain a cornerstone of effective model training. For businesses in the home services sector—especially locksmith services like Keymakr—the future promises numerous opportunities:
- Advanced Predictive Analytics: Enhanced machine learning algorithms with better data inputs can lead to highly precise predictive analytics.
- Real-Time Insights: With continuous data labeling and integration, businesses can gain real-time insights into customer behavior and preferences.
- Improved AI Applications: As artificial intelligence becomes more integral, businesses will leverage labeled data in increasingly sophisticated applications.
Conclusion
In conclusion, the significance of data labeling in machine learning cannot be overstated, especially for businesses like Keymakr operating in the home services and locksmith sectors. As data-driven decision-making becomes the norm, embracing effective data labeling strategies will not only elevate operational efficiency but also enhance customer satisfaction and loyalty. By implementing advanced data labeling techniques and leveraging the insights garnered, Keymakr can position itself as a leader in its industry, equipped to navigate the challenges of the future.
data labeling machine learning