Mastering the Art of Labeling Training Data
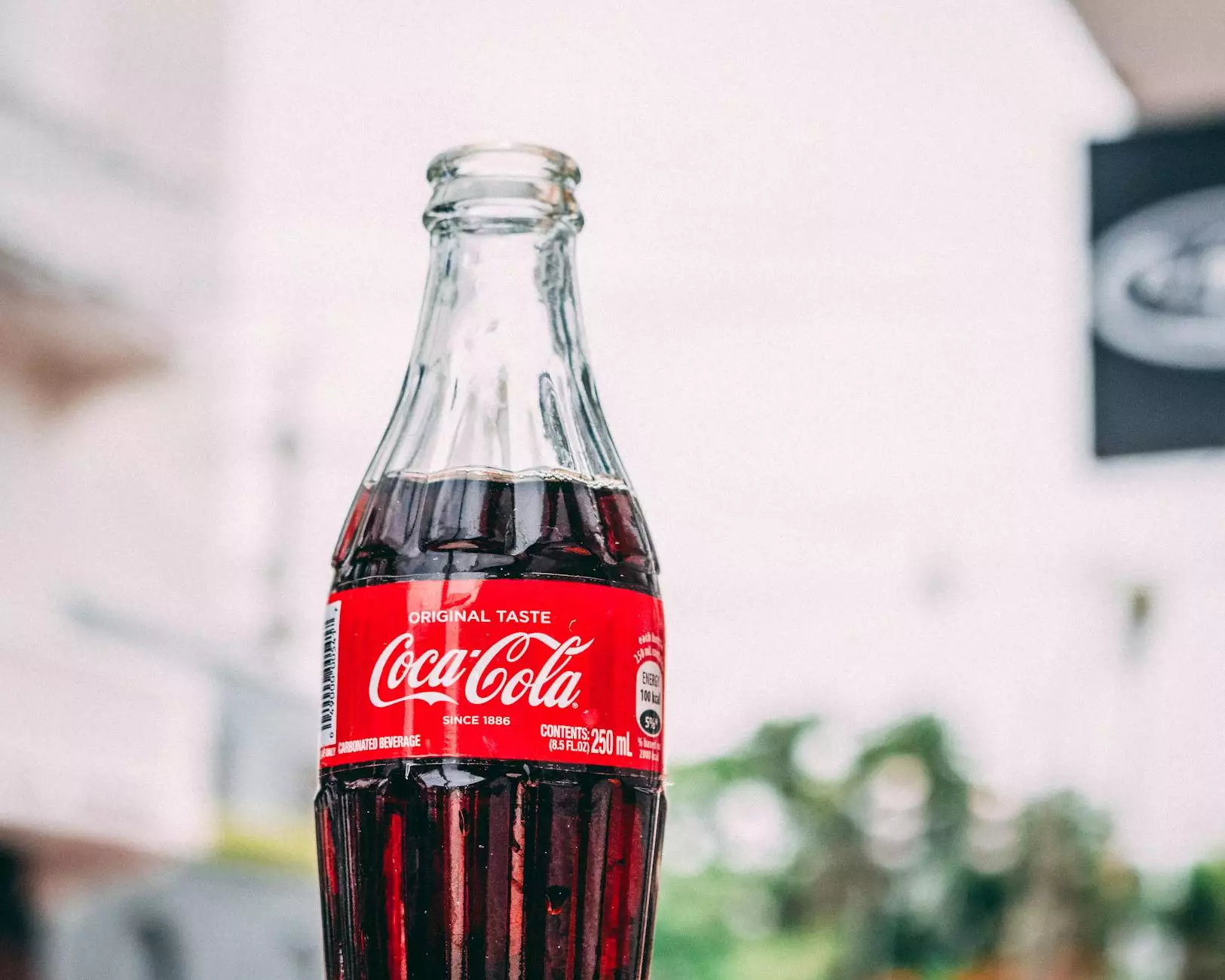
In the realm of artificial intelligence and machine learning, the process of labeling training data stands as a pivotal foundation for the success of any model. Accurate data annotation is essential for transforming raw data into actionable insights. At KeyLabs.ai, we recognize the challenges that come with effectively labeling training data and aim to provide innovative solutions through our comprehensive Data Annotation Tool and Data Annotation Platform.
Understanding the Importance of Labeling Training Data
Labeling training data involves the careful identification and categorization of information that machines need to learn and make predictions. It serves several critical functions, including:
- Enhancing Model Accuracy: High-quality labeled data results in better-trained models, which can significantly improve prediction accuracy.
- Facilitating Transfer Learning: Well-labeled datasets can be used to transfer knowledge across different but related tasks, speeding up the learning process.
- Improving Interpretability: Labeling provides context to the data, making it easier to understand how decisions are made by the algorithms.
- Driving Innovation: With accurate labels, businesses can unlock new functionalities and improve existing systems, fostering innovation.
Key Techniques in Labeling Training Data
To harness the power of labeling training data effectively, consider adopting the following techniques:
1. Define Clear Labeling Guidelines
Establishing comprehensive labeling guidelines is essential to maintain consistency across your dataset. Guidelines should cover:
- Definitions of each label/category.
- Examples of edge cases.
- Instructions on how to handle ambiguities.
These guidelines serve as a benchmark for annotators and help reduce subjectivity in labeling.
2. Utilize Automated Annotation Tools
Incorporating automation in your labeling process can enhance efficiency without compromising quality. KeyLabs.ai provides a Data Annotation Platform that leverages machine learning algorithms to:
- Perform semi-automated labeling, allowing annotators to validate and correct suggested labels.
- Speed up the data annotation process without losing the quality of labels.
- Create training data from existing datasets, reducing redundancy and conserving resources.
3. Implement Quality Assurance Processes
Systematic quality checks are vital to ensure the integrity of labeled data. Implement a quality assurance (QA) process that includes:
- Random Sampling: Regularly perform audits on random samples of labeled data to check for accuracy.
- Peer Review: Encourage collaboration among annotators to review each other’s work and provide feedback.
- Continuous Training: Offer regular training sessions for annotators to keep them updated on best practices and guidelines.
Challenges in Labeling Training Data
While labeling training data can bolster machine learning outcomes, various challenges may arise:
1. Data Diversity
Datasets often contain a variety of data points, causing inconsistencies in labeling. Ensuring diversity in your annotated data helps create more robust models and reduces bias.
2. Handling Ambiguities
Many data points may be ambiguous or have multiple potential labels. Establishing clear guidelines helps in addressing these situations, but there will always be a degree of subjectivity involved.
3. Scalability
As the volume of data grows, managing the labeling process can become overwhelming. Automated tools like those offered by KeyLabs.ai help businesses manage scaling their annotation efforts efficiently.
Advantages of Using KeyLabs.ai for Labeling Training Data
By opting for KeyLabs.ai's Data Annotation Tool and Data Annotation Platform, businesses can reap numerous benefits, including:
- Streamlined Workflow: Our platform enhances the labeling workflow, making it faster and less prone to errors.
- Cost-Effectiveness: Enable your team to focus on high-value tasks while automating time-consuming aspects of data annotation.
- Scalability: Easily scale your annotation efforts in alignment with project demands.
- Expertise and Support: Gain access to a team of experts who can assist with your specific labeling needs and challenges.
Best Practices for Effective Labeling Training Data
To further enhance your labeling training data process, consider integrating these best practices:
1. Engage Domain Experts
Involve domain experts in the labeling process to ensure that the data is correctly annotated. Their insights can greatly refine the quality of labels, especially in specialized fields like medicine or legal.
2. Use Iterative Labeling Approaches
Implement an iterative approach to labeling where initial labels are followed by rounds of adjustments based on QA feedback. This allows for the continuous improvement of data quality.
3. Collect Feedback from Machine Learning Models
After deploying models, utilize their performance feedback to inform your labeling process. If certain labels lead to frequent misclassifications, adjust accordingly.
Future Trends in Labeling Training Data
The landscape of labeling training data is continually evolving. Here are some trends to watch for:
1. Increased Use of AI for Data Annotation
As AI technology progresses, more sophisticated tools will emerge, making it possible to automate larger portions of the labeling process while maintaining high accuracy.
2. Crowdsourcing for Data Annotation
Leveraging crowdsourcing can expand the pool of annotators, allowing for rapid data labeling that can still meet quality standards through feedback loops and QA processes.
3. Enhanced Visualization Tools
Tools that allow annotators to visualize complex data (like images and videos) will help improve labeling accuracy and efficiency.
Conclusion: Elevate Your AI Initiatives with KeyLabs.ai
The significance of quality labeling training data cannot be understated in the success of machine learning models. KeyLabs.ai stands at the forefront of providing comprehensive solutions for data annotation, facilitating seamless integration of high-quality labeled data into your projects. By utilizing our advanced Data Annotation Tool and Data Annotation Platform, organizations can not only navigate the complexities of data labeling but also harness robust datasets that inform and drive innovation.
To learn more about how KeyLabs.ai can transform your data annotation projects and enhance your machine learning capabilities, please visit our website at KeyLabs.ai today!