Machine Learning Data Labeling: Unlocking the Future of AI
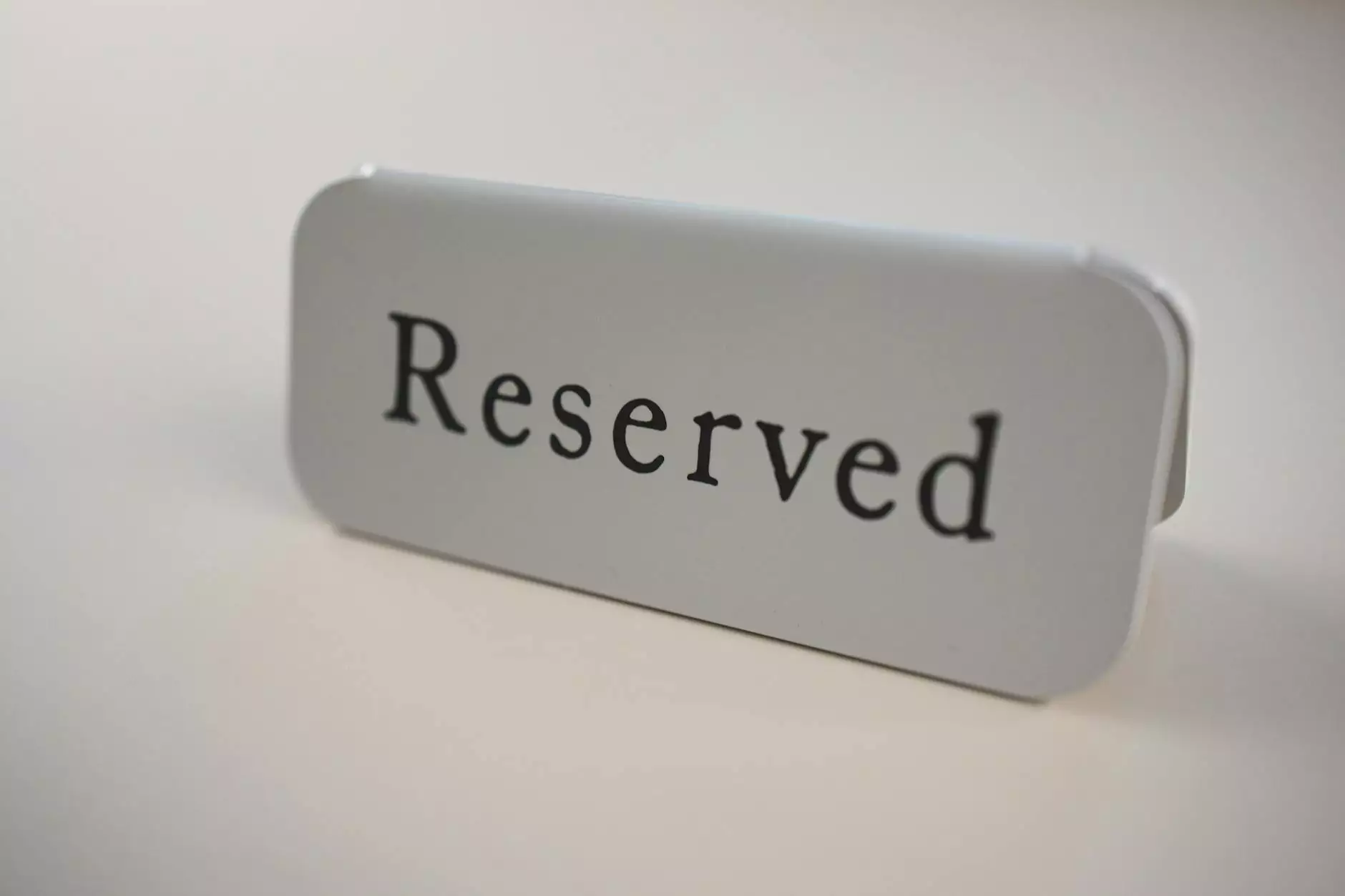
Machine learning data labeling is a crucial process that ensures the efficiency and effectiveness of artificial intelligence systems. In today's fast-paced digital world, businesses are increasingly relying on AI technologies to enhance their operations. From automating mundane tasks to improving decision-making processes, the applications of AI are vast. However, the success of any machine learning model is heavily reliant on the quality of the data used to train it. This is where data labeling comes into play.
Understanding the Importance of Data Labeling
Data labeling refers to the process of annotating data with relevant information to make it understandable for machine learning models. The labeled data serves as a training foundation, enabling algorithms to learn patterns, make predictions, and classify new data accurately. Here's why data labeling is essential:
- Quality Training Data: High-quality labeled data is fundamental for developing reliable algorithms. Poorly labeled data can lead to biased or incorrect predictions.
- Enhancing Model Accuracy: The accuracy of machine learning models improves significantly with properly labeled data. This leads to better performance in real-world applications.
- Facilitating Real-World Applications: AI applications in vision, speech, and text require comprehensive data to function correctly, further emphasizing the role of data labeling.
Types of Data That Require Labeling
In the realm of machine learning, various types of data require labeling, including:
- Images: Object recognition, facial recognition, and image classification require images to be annotated with bounding boxes or key points.
- Text: Natural language processing (NLP) involves labeling text data for sentiment analysis, entity recognition, and topic classification.
- Audio: Speech recognition systems need audio data to be labeled for transcription and emotion detection.
The Process of Machine Learning Data Labeling
The process of machine learning data labeling can be broken down into several key stages:
- Data Collection: Gathering diverse datasets that represent the problem space accurately.
- Data Preprocessing: Cleaning and organizing the data to ensure it is suitable for labeling.
- Annotation: Utilizing tools and platforms to label the data accurately.
- Quality Assurance: Implementing checks to ensure that the labeling is consistent and correct.
- Feedback and Iteration: Gathering feedback and re-adjusting labeled datasets to further improve the labels.
Choosing the Right Data Annotation Tool
Selecting the appropriate data annotation tool can significantly impact the speed and quality of the labeling process. Here are some key features to consider when evaluating data annotation tools:
- User-Friendly Interface: The tool should be intuitive and easy to navigate, allowing annotators to focus on their task rather than struggling with the software.
- Scalability: As projects grow, the tool should be able to handle larger datasets without compromising performance.
- Collaboration Features: Look for tools that facilitate collaboration among teams, allowing multiple users to work on projects simultaneously.
- Integration Capabilities: The tool should seamlessly integrate with other software solutions used in your AI development process.
- Customizable Options: The ability to customize labeling configurations according to specific project requirements is vital.
Keylabs.ai: Leading the Way in Data Annotation
At Keylabs.ai, we understand the intricacies of machine learning data labeling. Our Data Annotation Platform leverages state-of-the-art technology to streamline the labeling process, ensuring high-quality outputs tailored to your project needs.
Here are some features that make Keylabs.ai an industry leader:
- Advanced Annotation Tools: Our platform includes tools that support various data types, such as images, text, and audio, enabling comprehensive labeling capabilities.
- Expert Annotators: We employ a team of skilled annotators who are trained to deliver accurate and consistent labeling for optimal machine learning performance.
- Quality Control Mechanisms: Our quality assurance processes minimize errors and enhance the overall integrity of your datasets.
- Flexible Pricing Models: Keylabs.ai provides competitive pricing options tailored to meet diverse project budgets, ensuring accessibility for businesses of all sizes.
Benefits of Using Keylabs.ai for Your Data Annotation Needs
Choosing Keylabs.ai for your data annotation means unlocking several advantages:
- Increased Efficiency: Our platform optimizes the annotation workflow, allowing projects to progress faster.
- Improved Model Performance: With our high-quality labeled data, your machine learning models will perform better in real-world applications.
- Expert Support: Our support team is always available to assist you through the annotation process, ensuring a smooth experience.
- Data Security: We prioritize the security of your data, implementing robust measures to protect sensitive information.
Challenges in Machine Learning Data Labeling
While data labeling is essential, several challenges can arise during the process:
- Scalability: As datasets grow, the labeling process can quickly become overwhelming if not managed properly.
- Consistency: Ensuring that all annotators apply the same standards can be difficult, particularly with complex labeling tasks.
- Time Constraints: Meeting deadlines while maintaining quality can create pressure that impacts the annotation process.
Overcoming Data Labeling Challenges
To mitigate these challenges, consider the following strategies:
- Implement Automation: Integrating AI-assisted tools can help speed up the labeling process.
- Regular Training: Continuously educate your annotators to ensure consistency and accuracy across projects.
- Utilize Feedback Loops: Establish regular feedback sessions to address quality issues and improve processes over time.
Future of Machine Learning Data Labeling
As technology evolves, the landscape of machine learning data labeling is also set to change. Key trends to watch include:
- Increased Automation: The rise of AI-powered annotation tools will allow for faster and more efficient labeling processes, while still maintaining high standards.
- Integration of Crowdsourcing: Crowdsourced annotation can provide a diverse range of perspectives, enhancing the quality of labeled data.
- Focus on Ethical AI: As awareness of bias in AI grows, the demand for ethically labeled data will become more prominent.
Conclusion
The significance of machine learning data labeling cannot be overstated. It is a foundational aspect of developing robust AI systems that can solve real-world problems. With the right tools, such as those offered by Keylabs.ai, businesses can ensure that their machine learning projects are rooted in high-quality labeled data. This not only enhances model accuracy but also propels organizations toward achieving their AI goals with confidence and efficiency. Embrace the future of AI with powerful data annotation solutions that pave the way for innovation and success.